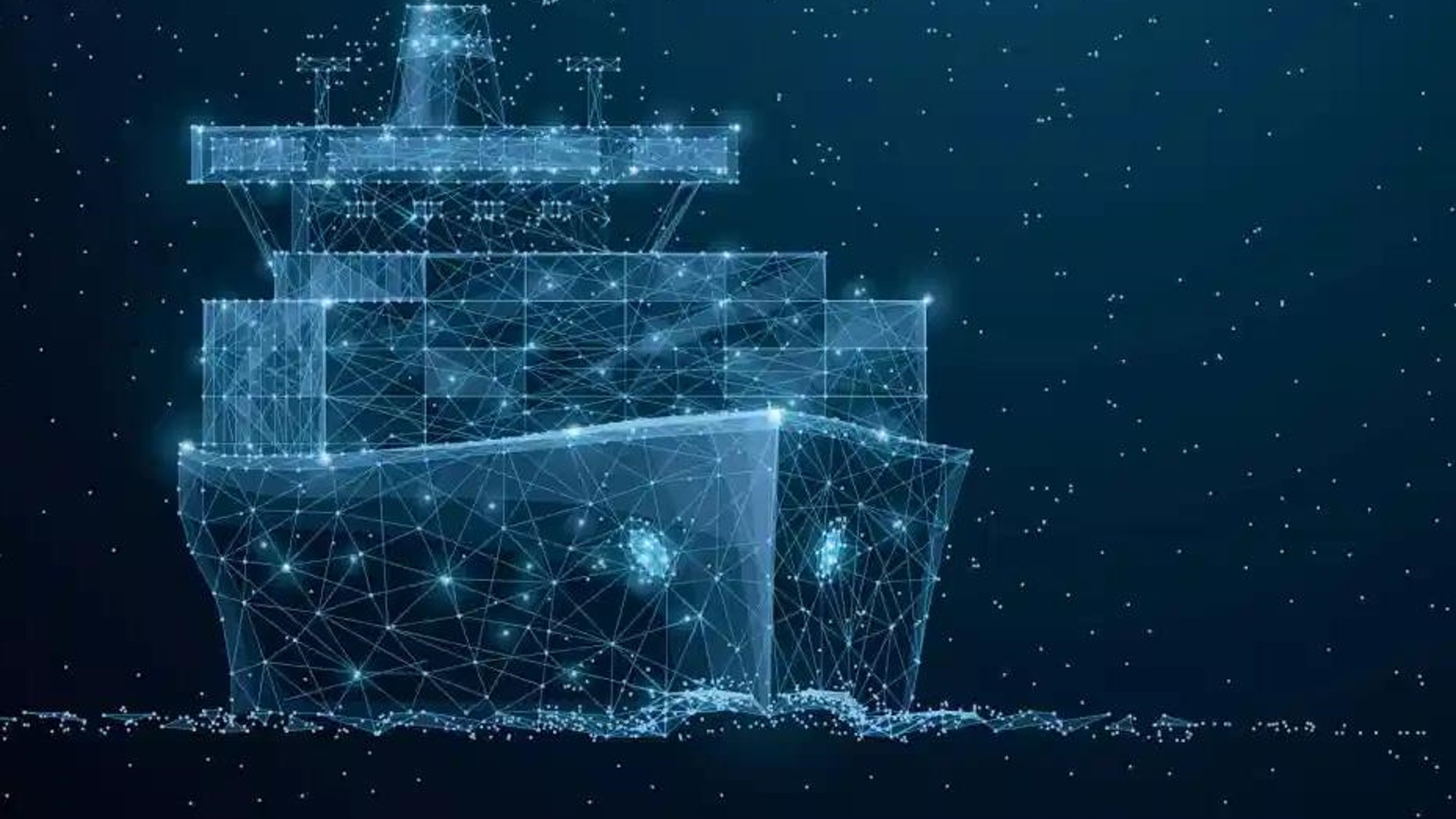
Future-oriented maritime services will increasingly rely on the intelligent use of data from maritime supply chains, transport markets as well as fleet management and port operations. The comprehensive availability of digital data in real time, seamless data exchange and systematic analysis through machine learning technologies as a basis of data-driven decision making can lead to significant changes in the operating principles and business logic of maritime logistics.
Machine learning is considered a key technology for the optimization of business processes in maritime logistics and shipping. No doubt, it offers opportunities to improve cost-efficiency, safety and sustainability of transporting goods in maritime supply chains. While the excitement about machine learning continues to grow, far-reaching promises about the disruptive potential of data-based innovations are widespread, and it can sometimes be difficult to distinguish fact from fiction.
The white paper »Machine Learning in Maritime Logistics« attempts to clear the fog around artificial intelligence (AI) in maritime logistics and shed some light on the buzzword machine learning.
“Exploiting the value of data with machine learning is increasingly important for gaining and maintaining a competitive advantage in all segments of the maritime industry.”
The main inputs and contributions are:
- Data analytics provides a powerful set of tools but the underlying data must be representative of the problem and data sets must be sufficiently large. For many business use cases, creating or obtaining such data sets can be difficult. Moreover, insufficient data quality is a related challenge that companies have to address. In consequence, a large part of the time and effort in data driven projects is actually spent on collecting, structuring and storing data as well as cleaning and preprocessing it.
- Before getting started with machine learning projects in practice, it is helpful to take a step back and define a structured and systematic approach for achieving a specific project goal. Advancing digitalization and the increasing data availability have also given rise to new project management models that take into account the specific requirements of data-based projects.
- Although companies have proven to be successful when making decisions based on experience and «gut instinct», the value of data-based decision making is undoubtedly agreed upon and an important factor to improve performance. It would hardly be possible, for example, to reach todays levels of process automation without utilizing data.
- Data-driven decision-making provides powerful solutions to advance from a system based primarily on intuition, experience and ad-hoc processes. This does not mean that it is the best solution for every case or an all-or-nothing approach replacing experience and domain knowledge. On the contrary, the goal is to design and implement data-driven decision-making systems which complement expert knowledge and enlarge the horizon of the decision maker.
- By combining data from different sources in supply chains, transparency can be increased significantly and a single source of clean and pre-processed data allows for predictive insights. In this context, data-driven decision-making assumes the function of combining, analysing and visualizing data from different sources and providing it as an input utilizing methods from different areas as data science, ML and advanced analytics.
- Decision-making in maritime logistics often involves external, fluctuating factors that are stochastic in nature and therefore complicate planning and identifying well-considered actions. This underlines the importance of reliable forecasts for decisions under uncertainty. With machine learning-based predictive analytics methods, forecasts can reach a new level of accuracy and reliable predictions for business-relevant influencing factors are achievable.
The goal is to design and implement data-driven decision-making systems which complement expert knowledge and enlarge the horizon of the decision maker.
[button url=»https://www.cml.fraunhofer.de/en/press/studies/white-paper–machine-learning-in-maritime-logistics-.html» class=»» bg=»» hover_bg=»» size=»14px» color=»» radius=»0px» width=»0px» height=»0px» target=»_self»] See the complete paper [/button]
Machine Learning in Maritime Logistics
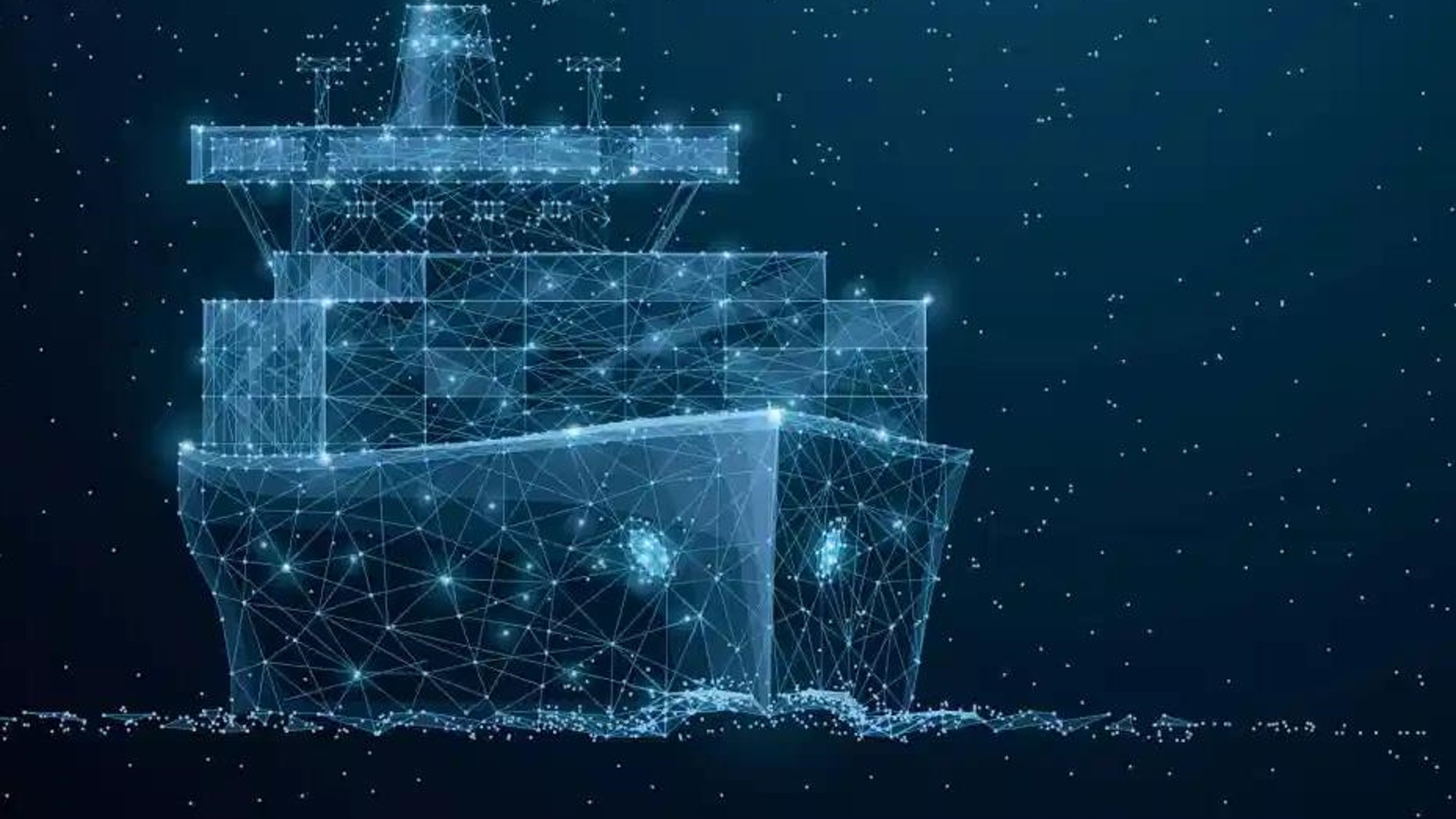
Future-oriented maritime services will increasingly rely on the intelligent use of data from maritime supply chains, transport markets as well as fleet management and port operations. The comprehensive availability of digital data in real time, seamless data exchange and systematic analysis through machine learning technologies as a basis of data-driven decision making can lead to significant changes in the operating principles and business logic of maritime logistics.
Machine learning is considered a key technology for the optimization of business processes in maritime logistics and shipping. No doubt, it offers opportunities to improve cost-efficiency, safety and sustainability of transporting goods in maritime supply chains. While the excitement about machine learning continues to grow, far-reaching promises about the disruptive potential of data-based innovations are widespread, and it can sometimes be difficult to distinguish fact from fiction.
The white paper »Machine Learning in Maritime Logistics« attempts to clear the fog around artificial intelligence (AI) in maritime logistics and shed some light on the buzzword machine learning.
“Exploiting the value of data with machine learning is increasingly important for gaining and maintaining a competitive advantage in all segments of the maritime industry.”
The main inputs and contributions are:
- Data analytics provides a powerful set of tools but the underlying data must be representative of the problem and data sets must be sufficiently large. For many business use cases, creating or obtaining such data sets can be difficult. Moreover, insufficient data quality is a related challenge that companies have to address. In consequence, a large part of the time and effort in data driven projects is actually spent on collecting, structuring and storing data as well as cleaning and preprocessing it.
- Before getting started with machine learning projects in practice, it is helpful to take a step back and define a structured and systematic approach for achieving a specific project goal. Advancing digitalization and the increasing data availability have also given rise to new project management models that take into account the specific requirements of data-based projects.
- Although companies have proven to be successful when making decisions based on experience and «gut instinct», the value of data-based decision making is undoubtedly agreed upon and an important factor to improve performance. It would hardly be possible, for example, to reach todays levels of process automation without utilizing data.
- Data-driven decision-making provides powerful solutions to advance from a system based primarily on intuition, experience and ad-hoc processes. This does not mean that it is the best solution for every case or an all-or-nothing approach replacing experience and domain knowledge. On the contrary, the goal is to design and implement data-driven decision-making systems which complement expert knowledge and enlarge the horizon of the decision maker.
- By combining data from different sources in supply chains, transparency can be increased significantly and a single source of clean and pre-processed data allows for predictive insights. In this context, data-driven decision-making assumes the function of combining, analysing and visualizing data from different sources and providing it as an input utilizing methods from different areas as data science, ML and advanced analytics.
- Decision-making in maritime logistics often involves external, fluctuating factors that are stochastic in nature and therefore complicate planning and identifying well-considered actions. This underlines the importance of reliable forecasts for decisions under uncertainty. With machine learning-based predictive analytics methods, forecasts can reach a new level of accuracy and reliable predictions for business-relevant influencing factors are achievable.
The goal is to design and implement data-driven decision-making systems which complement expert knowledge and enlarge the horizon of the decision maker.
[button url=»https://www.cml.fraunhofer.de/en/press/studies/white-paper–machine-learning-in-maritime-logistics-.html» class=»» bg=»» hover_bg=»» size=»14px» color=»» radius=»0px» width=»0px» height=»0px» target=»_self»] See the complete paper [/button]
0 respuestas en "Machine Learning in Maritime Logistics"
Deja un mensaje
Lo siento, debes estar conectado para publicar un comentario.
0 respuestas en "Machine Learning in Maritime Logistics"